5 Pain Points of Market Research
The Big, Scary Problem
Running a business is like playing a game. Except it’s against a huge, complex, dynamic system where all the players in the game (businesses and customers) make decisions constantly, and these decisions impact one-another in near real time. So, playing this game well necessitates ~real-time sources of data and feedback from customers.
Companies, and specifically marketers, must make countless decisions regularly. In product marketing, people are constantly deciding how to modify existing products/lines to meet customer needs, which new products to build, how to position products, etc.
To make these decisions, businesses look to understand their customers deeply. How? People look to traditional market research and testing, from qualitative methodologies like ethnographies and focus groups to surveys to quantitative methods and control trials, etc — using these techniques to quantify and disentangle the forces that impact customer perception and decisions.
However, the truth is, the processes for running traditional research are complex, poorly validated, slow, and worst of all, results are usually short-lived. Here are the biggest challenges our prospects face.
1. No Real Validation
Market research and testing approaches have been used for decades without any real validation. They’re often used to predict how a product, service, or campaign may perform in a market, but the resulting performance of said asset is not used to evaluate the prediction. There’s a good reason for this, attribution: attributing the success or failure of a product/campaign to a set of decisions is a very hard problem to solve.
In the absence of fast, digital feedback loops (say in the case of performance marketing), there’s usually no way of knowing the validity of outcomes from traditional market research approaches.
2. The Bias of Human Participants
One way to try and understand what specifically drives customer behavior and perception is by asking people these questions directly through methods such as focus groups, panels, surveys, etc. There are two big problems with this approach:
1) People often don’t know exactly how or why they made a particular decision, so our self-recalled accounts can be heavily biased or too simplistic.
Imagine someone asks you why you buy a particular type of cereal. Now, you’ve been buying this cereal brand for ages - so it’s hard to recall why exactly you like this cereal. Maybe it’s because you associate the brand with health, or maybe the cereal reminds you of your childhood. It could be a whole complex set of reasons that you don’t understand; but if you’re answering this question in a survey, you may be inclined to just simply say “because it tastes good”.
2) People are highly susceptible to fatigue when answering questions thoughtfully. This makes it difficult to scale traditional methods.
The self-reporting and fatigue biases that arise from directly asking people questions about behavior and decision-making can lead to a deeply flawed dataset.
3. The Slowness (and then Staleness) of Acquiring Information
Acquiring high quality data through market research can be incredibly slow, often taking weeks or months to obtain science-backed signals. Furthermore, running research with a high quality bar can mean $10k - $Ms in cost, from recruiting to compensating participants and moderators at scale.
While research is expensive and slow, it’s further complicated by the fact that customer behavior and perception is constantly changing. Remember how we described markets as big, complex dynamic systems where all players constantly make decisions that influence each other? This means that markets are always evolving, and when they do, signals that we gathered through research become stale. Data from research can become less useful in a matter of days and weeks, and completely useless in months and years. So, current market research solutions are error prone by nature.
4. Data are Easy to Manipulate
Just as statistics can be misconstrued and manipulated to tell conflicting stories, insights from market research and testing can easily be used in a leading way to get “desired answers”. This is because the validity of results is almost impossible to measure, and all related variables cannot be perfectly controlled. So we can easily misconstrue correlations, coincidences, or biases as valuable information. Especially given that a lot of these approaches are used in corporate environments with tight deadlines and high-stakes business decisions.
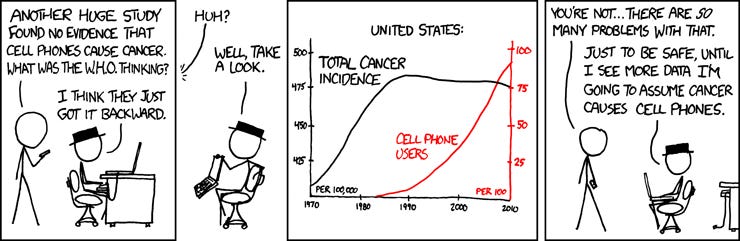
5. Lose the Details, Dull the Value
Running traditional research usually means simplifying learning outcomes to ask questions in ways people can actually answer. This simplification, or removal of details, can massively dilute the value and effectiveness of a lot of research methodologies. For example, consider someone buying something in a retail store. Their purchase decision could be influenced by a number of things – such as associations between the brand and a particular feeling, recalling a fond memory, their feeling in that specific moment, etc.
This kind of detailed context is very difficult to describe in traditional research. The real insights are detailed, nuanced, and usually entangled with context. So when we turn a complicated customer decision into a simple survey question (for example, “how likely are you to purchase the product”?), how effective can this really be at getting useful signals for our specific business problems?
A Breakthrough Solution
So it’s no surprise that current methods for market research and asset/concept testing are far from perfect. But we use these methods because we don’t have better alternatives, and making high stakes product, marketing, and creative decisions in a vacuum is not an option.
To get better outcomes, we believe in iterative, near real-time sources of information that use science-backed methodologies to measure, model, and predict customer behavior.
This is the reason we founded Keplar - a customer simulation platform that allows you to get instant insights, feedback, and ideas through data-driven predictive simulations of your target customers. We do this by leveraging generative models to process massive amounts of text, image, and video data, to build a science-backed model of perception and association. We go beyond insights to deliver actionable results with enterprise-safe automations. All at a dramatically improved speed and cost to traditional research and testing.
That’s what we’re building at Keplar. Check us out!